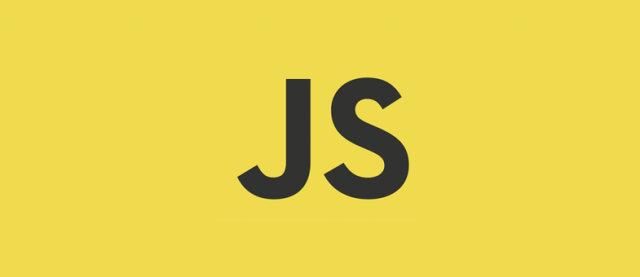
【JS】画像のリアルタイム物体検出 数行で実装 デモ有 TensorFlow.js coco-ssd
2018-12-1813 min read
目次
概要
TensorFlow.js+coco-ssdを用いて、1枚の画像から複数のオブジェクト検出を行う方法を紹介します。 デモも作ったので興味があれば触ってみてください。
オブジェクト検出
オブジェクト検出にはTensorFlow.js + coco-ssdを利用しました。
https://www.npmjs.com/package/@tensorflow-models/coco-ssd
試す
いくつかの画像で試してみました。
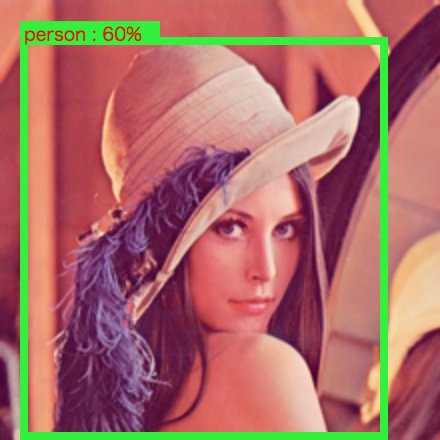
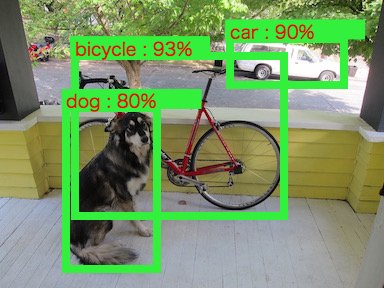
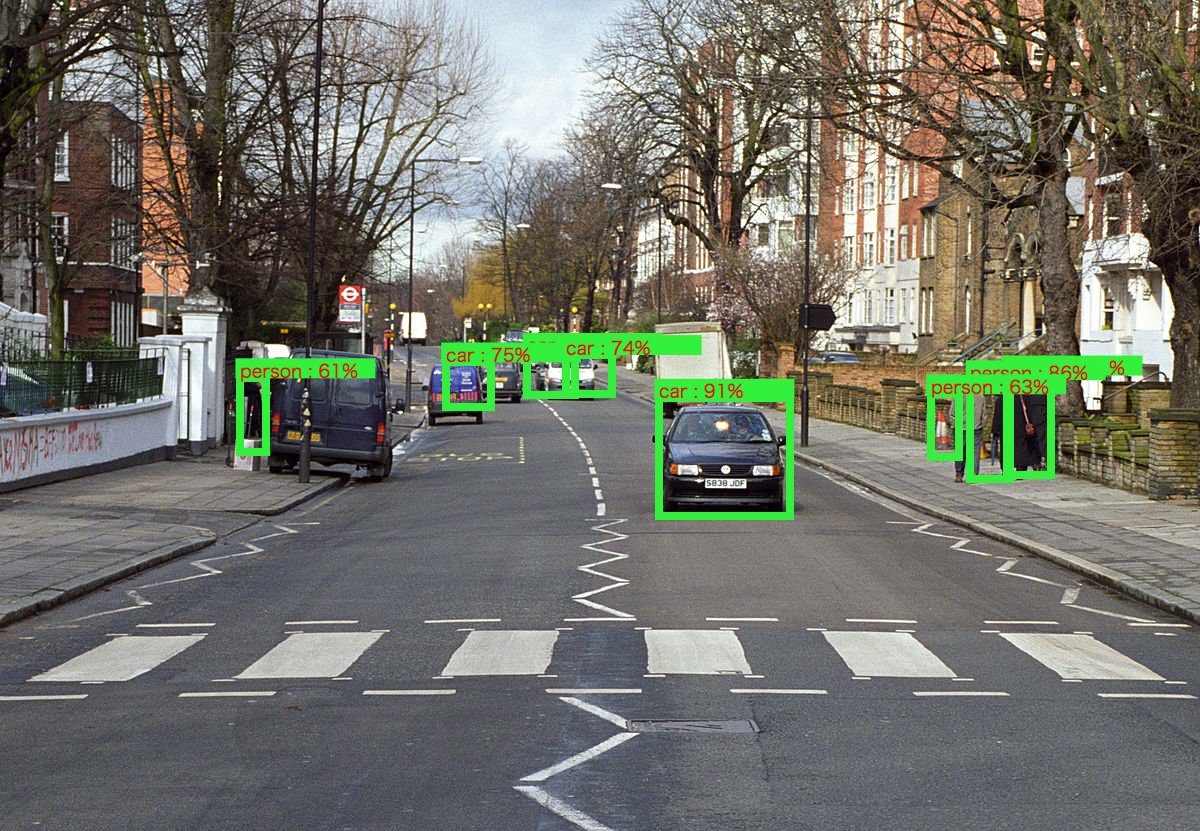
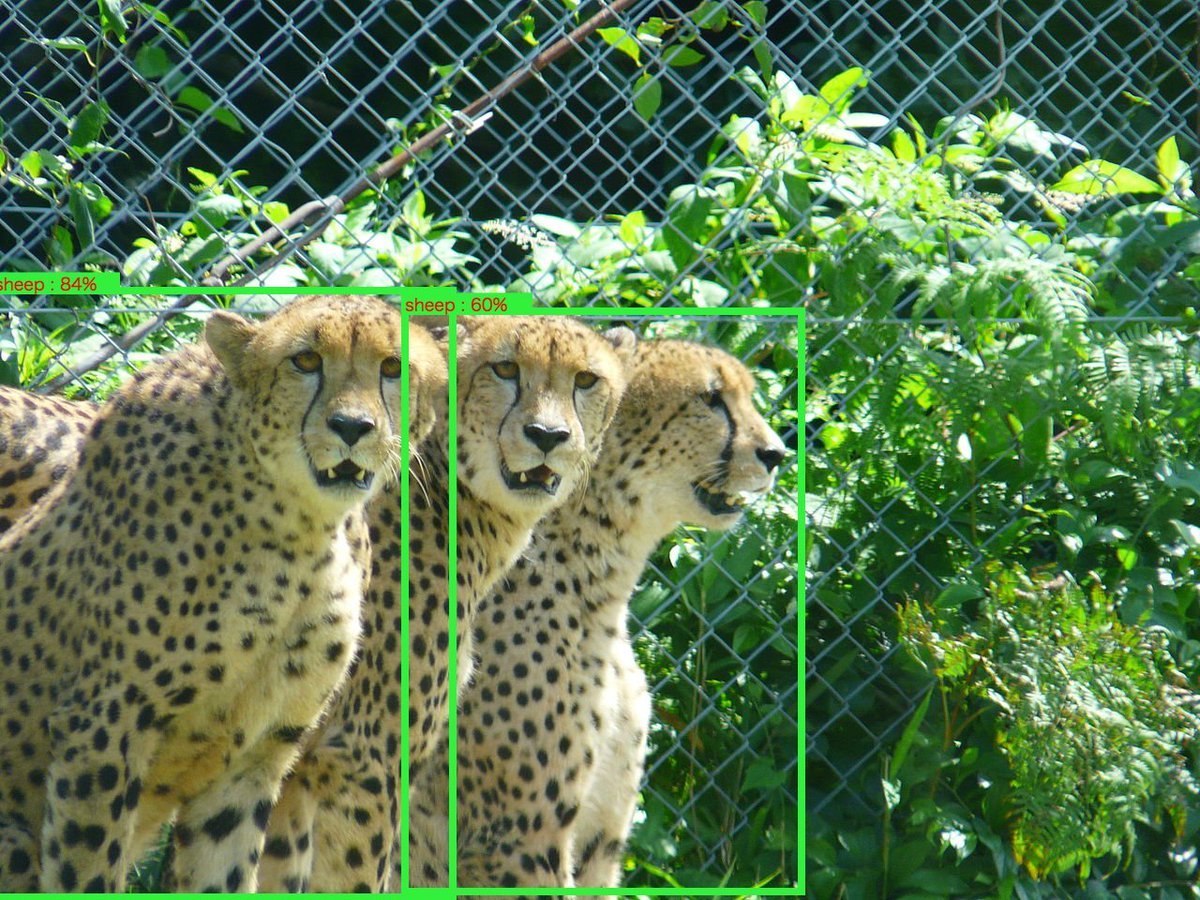
サンプルコードと解説
scriptタグで始める
単純にscriptタグで始めるのであれば、以下のコードで始めることができます。
<script src="https://cdn.jsdelivr.net/npm/@tensorflow/tfjs"> </script>
<script src="https://cdn.jsdelivr.net/npm/@tensorflow-models/coco-ssd"> </script>
<img id="img" src="test.jpg">
<script>
cocoSsd.load().then(model => {
model.detect(img).then(predictions => {
console.log('Predictions: ', predictions);
});
});
</script>
coco-ssd API
cocoSsd.load();
このメソッドでモデルをロードします。引数を渡さない場合は”lite_mobilenet_v2”が選択されます。
model.detect();
model.detect()で物体検出を行います。引数にはhtmlのimg要素canvas要素、ImageDataオブジェクトを渡すことができます。 物体の検出に成功した場合は次のJSONのような形で値が渡されます。 失敗した場合はからの配列が渡ってきます。この値は人を検出した時の例です。
[
{
"bbox": [
225.4661464691162,
21.420029640197754,
194.02139472961426,
326.7346258163452
],
"class": "person",
"score": 0.802341103553772
}
]
デモ
https://jsfiddle.net/s_yoshiki/tn7usb9p/show
デモのサンプルコード
このサンプルソースではcanvasを利用していますが、img要素などに置き換えることもできます。
<input type="file" id="file">
<canvas id="canvas"></canvas>
<pre id="debug">
ラベルとかの処理はcanvasのAPIを叩いて実装しています。
const canvas = document.getElementById('canvas');
document.getElementById('file').onchange = function() {
let img = this.files[0];
let reader = new FileReader();
reader.readAsDataURL(img);
reader.onload = function() {
detectObjects(reader.result);
};
};
function detectObjects(url) {
let ctx = canvas.getContext('2d');
let image = new Image();
let debug_dom = document.getElementById('debug');
image.src = url;
image.onload = () => {
canvas.width = image.width;
canvas.height = image.height;
ctx.drawImage(image, 0, 0);
cocoSsd.load().then(model => {
model.detect(canvas).then(predictions => {
for (var i = 0; i < predictions.length; i++) {
var obj = predictions[i];
var box = obj.bbox;
console.log(i);
drawRect(box[0], box[1], box[2], box[3]);
drawLabel(
obj['class']
+ ' : '
+ parseInt(obj['score'] * 100, 10)
+ '%',
box[0],
box[1],
);
}
debug_dom.innerHTML = JSON.stringify(predictions, null, '\t');
});
});
};
}
function drawRect(x, y, w, h) {
var ctx = canvas.getContext('2d');
ctx.beginPath();
ctx.rect(
parseInt(x, 10),
parseInt(y, 10),
parseInt(w, 10),
parseInt(h, 10),
);
ctx.strokeStyle = 'rgb(50, 240, 60)';
ctx.lineWidth = 8;
ctx.stroke();
ctx.closePath();
}
function drawLabel(text, x, y) {
var ctx = canvas.getContext('2d');
ctx.beginPath();
ctx.rect(x - 5, y - 20, 140, 20);
ctx.fillStyle = 'rgb(50, 240, 60)';
ctx.fill();
ctx.closePath();
ctx.beginPath();
ctx.font = '18px \'MS Pゴシック\'';
ctx.fillStyle = 'red';
ctx.fillText(text, parseInt(x, 10), parseInt(y, 10));
ctx.closePath();
}
参考
https://www.npmjs.com/package/@tensorflow-models/coco-ssd
https://hackernoon.com/tensorflow-js-real-time-object-detection-in-10-lines-of-code-baf15dfb95b2
Recommends
New Posts
Hot posts!
Date
Tags
Author